基于卷积神经网络与长短期记忆网络结合空间注意力机制的数据分类预测模型-Matlab 2020版及以上代码实现,基于卷积神经网络与长短期记忆网络的深度学习模型结合空间注意力机制在Matlab 2020
资源文件列表:

1.jpg 78.53KB
2.jpg 85.95KB
3.jpg 75.68KB
4.jpg 76.44KB
5.jpg 101.96KB
6.jpg 301.65KB
7.jpg 284.49KB
基于卷积神经网络和长短期记忆网络结合空间注意力.txt 2.31KB
基于卷积神经网络长短.html 1.34MB
基于卷积神经网络长短期记忆网络.html 1.34MB
基于卷积神经网络长短期记忆网络结合空.txt 2.39KB
基于卷积神经网络长短期记忆网络结合空间.txt 2.29KB
基于卷积神经网络长短期记忆网络结合空间注意力机制.html 1.34MB
基于卷积神经网络长短期记忆网络结合空间注意力机制的.doc 3.02KB
文章标题基于卷积神经网络长短期.txt 2.34KB
文章标题基于卷积神经网络长短期记忆网络.txt 2.27KB
资源介绍:
基于卷积神经网络与长短期记忆网络结合空间注意力机制的数据分类预测模型——Matlab 2020版及以上代码实现,基于卷积神经网络与长短期记忆网络的深度学习模型结合空间注意力机制在Matlab 2020版本及以上实现数据分类预测,基于卷积神经网络-长短期记忆网络结合空间注意力机制(CNN-LSTM-Spatial Attention)的数据分类预测 matlab代码,2020版本及以上 ,核心关键词:卷积神经网络; 长短期记忆网络; 空间注意力机制; 数据分类预测; MATLAB 2020版本及以上。,基于CNN-LSTM-Spatial Attention的数据分类预测Matlab 2020版代码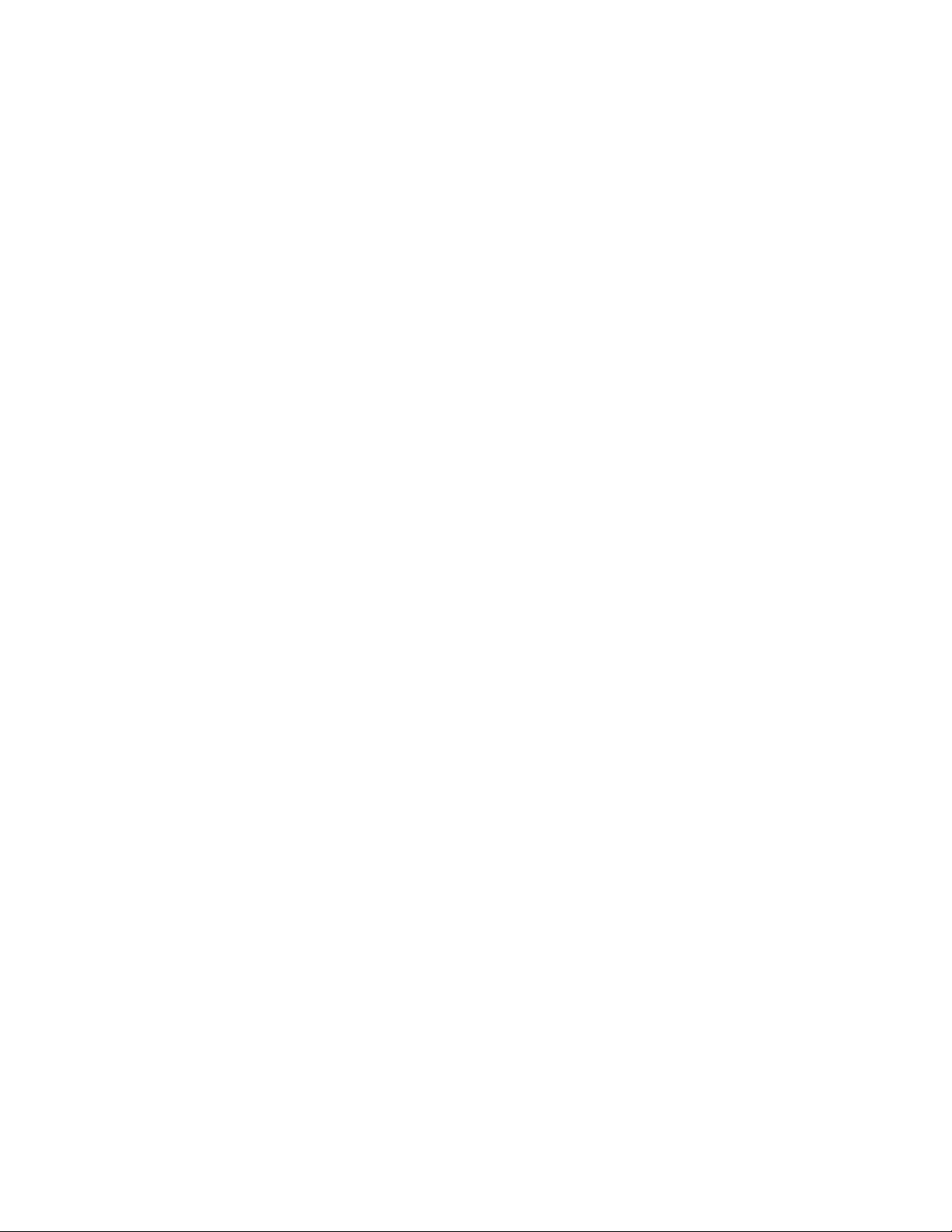
**基于卷积神经网络-长短期记忆网络结合空间注意力机制(CNN-LSTM-Spatial Attention)的
数据分类预测**
一、引言
随着深度学习技术的不断发展,卷积神经网络(CNN)和长短期记忆网络(LSTM)已经在多个领域展
现出强大的数据处理能力。然而,为了更好地捕捉数据的空间信息和时间依赖性,研究人员进一步结
合了空间注意力机制来提升模型的表现。本文将详细介绍基于 CNN-LSTM 结合空间注意力机制(
Spatial Attention)的数据分类预测方法,并使用 MATLAB 2020 版本及以上版本编写相关代码
。
二、方法论
1. 数据预处理
在进行模型训练之前,需要对原始数据进行预处理,包括数据清洗、归一化等步骤。
2. 构建 CNN-LSTM 模型
- 卷积神经网络(CNN):用于提取数据的空间特征。
- 长短期记忆网络(LSTM):用于捕捉时间序列数据中的时间依赖性。
- 空间注意力机制:通过注意力机制增强模型对重要空间特征的关注度。
3. 模型训练与优化
使用适当的损失函数和优化算法对模型进行训练,并根据实际需求调整超参数。
三、MATLAB 代码实现(示例)
以下是一个简化的 MATLAB 代码示例,用于构建和训练基于 CNN-LSTM 结合空间注意力机制的数据分
类模型。请注意,这只是一个基本框架,具体实现可能需要根据实际数据和需求进行调整。
```matlab
% 加载或预处理数据集(这里假设已经完成)
% 数据集应当是四维的,例如:输入特征[样本数, 时间步长, 特征维度, 空间维度]
% 或者需要进行适当的重塑以符合该格式。
% 定义网络结构
layers = [
imageInputLayer([时间步长 特征维度], 'Normalization', 'none') % 输入层
convolution2dLayer(卷积核大小, 'Padding', 'same') % 卷积层,根据需要调整卷
积核大小和填充方式